How to Become a Data Analyst With No Experience
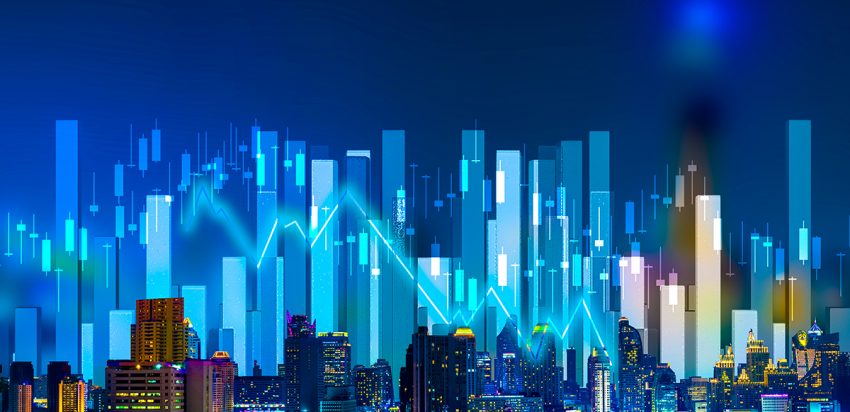
If you’re wondering how to become a data analyst, there’s a good chance that numbers appeal to you.
While seemingly endless data sheets might throw others off-balance, you find yourself drawn in, intrigued by the opportunity to parse those (almost) impenetrable walls of information for hidden insights and useful tips. Given our data-driven world, who could blame you for wanting to make a career out of your interest and talent?
In this article, we’ll walk you through how to be a data analyst and what you’ll need to do to hone your natural aptitudes into marketable analytical talents. We’ll also give you an idea of where your skills as a data analyst will take you during your career and how you can tailor your skill set to appeal to employers in the industry of your choice.
This article includes information on:
- Understanding Where You Want To Go
- Receiving Foundational Training
- Obtaining the Skills
- Breaking into Your Chosen Industry
Ready to learn how to become a data analyst? Let’s get started.
Understand Where You Want to Go
Before we start plotting a path for your career, it’s important that you gain a clear understanding of where you’ll be going and what you can expect to find when you get there.
What Is a Data Analyst?
A data analyst is a tech professional who helps businesses, nonprofits, and other organizations develop insights from countless facts and figures on a day-to-day basis.
These data-savvy professionals deploy a wide range of techniques in their work. These include but are not limited to programming, machine learning, mathematics, statistics, and data gathering to provide numbers-backed insights.
While the niche responsibilities that data analysts take on can vary across industries, companies, and jobs, most professionals spend their workdays combing through enormous databases for trends, patterns, or other observations. These observations allow them to develop informed opinions on recent and long-term trends, make predictions, and communicate their insights with company stakeholders.
Data analysts are particularly valuable to businesses because they can identify trends and create predictive models before problems — or costly strategic mistakes — occur. Data analysts can also help companies gauge whether products and services are likely to be successful by assessing the performance of ads, platforms, and business site pages among target audiences.
Data analysts are often associated with the tech sector; however, they are by no means limited to it. In fact, data analysts can land roles at large investment banks, private equity firms, medical technology companies, and even community nonprofits.
Today, virtually every modern business has access to a wealth of data — so much so that their collected troves are impossible to use without experienced professionals. Thanks to data analysts with a clear understanding of how to deploy data-driven insights, however, the benefits of collecting those information troves are hard to understate.
In 2018, the business intelligence firm Microstrategy polled companies from a variety of industries and across the globe on their utilization of data. Their researchers reported that 60 percent were using data analytics to boost process and cost efficiency, 57 percent were applying it to drive strategy and change, and 52 percent relied on data analysts to monitor and improve financial performance.
Given these benefits and our increased reliance on both data and the insights it offers, it should be no surprise that data analysts are in high demand. According to a 2019 report from Indeed, the job postings for data scientists has risen a whopping 31 percent year-over-year.
“Before, data science jobs were mostly constrained to the tech and finance sectors,” Linkedin Learning analyst Paul Patrone wrote of the need for talent. “Now, virtually every industry, from retail to manufacturing, is collecting data on their customers. That’s causing a surge of demand for data scientists who can best interpret all that data. And yet, since it’s a relatively new job, few professionals possess that skill set. Hence, most American cities, instead of just finance- and tech-heavy ones like San Francisco and New York, are short data scientists.”
Wondering where you might end up when you become a data analyst? Here are a few of the most data-dependent industries:
- Retail: Retail is a fiercely competitive industry, so companies that fail to correctly identify new trends risk falling behind their competitors and out of consumer favor. With well-deployed data analytics, retailers can anticipate what their customers will want — and then provide it.
- Finance: The banking industry utilizes predictive analytics to bolster customer service, improve efficiency, and limit operational issues such as fraud.
- Manufacturing: Data analysts employed in manufacturing often use predictive analytics to assess the probability of system failure and schedule repairs before any machines break down and impact production schedules.
- Healthcare: With predictive analytics, healthcare organizations can gain a comprehensive understanding not only of how their facilities are used but also how to optimize their existing resources. This capability is invaluable, as it allows providers to streamline patient service and provide the best care to the most patients possible.
- Transportation: Organizations in this field can parse collected data to analyze how people are likely to use transportation services and identify increased risk for problems such as excessive traffic, collisions, or demand. These predictive insights empower transportation officials to tweak travel schedules and services and ensure that all transit runs smoothly.
Of course, we’re starting to get ahead of ourselves. Let’s start by answering the more immediate question: How can I become a data analyst?
Receive the Foundational Training
If you ask anyone in the field for advice on how to get into data analytics, their immediate answer will almost always be the same: education and training.
Regardless of the industry that they eventually find themselves working in, all data analysts need to obtain a core set of skills. Let’s review a few of the foundational competencies you’ll need to acquire before you ever step into the hiring pool.
Fundamental Statistics
It’s hard to get very far in data analytics without some familiarity with statistical thinking and theory, especially if you intend to specialize in machine learning. Having a basic grasp on statistical principles will improve your ability to classify information, create understandable data visualizations, and use advanced data-sampling techniques.
Python
Python is one of the most popular programming languages in data analytics. Analysts use pre-installed modules and a vast collection of libraries to retrieve, clean, and visualize data, as well as build analytical models.
Excel
You may know the basics of Excel, but most people don’t know quite how advanced spreadsheet analysis can get. Not only is this staple office software intuitive, flexible, and collaborative — it’s incredibly useful.
Data analysts can import information from external databases and then use internal Excel features such as pivot tables and VBA scripting to perform quick analyses and generate easy-to-understand tables and reports.
SQL/NoSQL
Both SQL and NoSQL are languages used to communicate with databases. The difference between the two lies in the type of databases each language can manipulate.
SQL, or Structured Query Language, is the standard language used to communicate with relational databases — i.e., those that store related data points according to their connections. It can be used to update, retrieve, or delete information from those databases.
NoSQL, as the name suggests, is often used to query non-relational databases. Though data analysts should be familiar with both languages, they should prioritize learning NoSQL. Non-relational databases (and NoSQL, by extension) don’t face the restrictions posed by a relational structure and are thus well-suited to handling large data sets. This capability makes them popular among the many businesses that collect large quantities of information — and are therefore increasingly relevant to aspiring data analysts.
Hadoop
Hadoop is a popular open-source software that provides massive amounts of storage for any type of data. The framework can accommodate concurrent tasks, making data analysis much less time- and resource-intensive.
Tableau
Tableau is a helpful tool that allows analysts to isolate data according to different factors; this enables analysts to home in on particular regions, demographics, or outliers to develop a better understanding of the data set.
Front End Development Skills
Most data analysts need to communicate their findings to clients, other departments, or company stakeholders. CSS and HTML can help analysts create pleasing online platforms that host their data-filled visual representations.
HTML, or HyperText Markup Language, is used to describe the underlying structure of a web page. Developers can use defining “tags” to place images, paragraphs, input forms, and other features onto a site.
CSS (Cascading Style Sheets) describes how HTML elements should be styled, affecting font sizes, colors, spacing, and other design concerns.
How to Obtain These Skills
It’s a seemingly simple question: How do I become a data analyst?
But the answer is complicated. There is no “right” path into analytics. There are several ways to accumulate the skills you’ll need to thrive professionally; you might invest in a four-year college degree, enroll in a shorter boot camp program, teach yourself via self-study, or do some combination of the three. To employers, how you obtain your skills doesn’t matter as much as the fact that you have them.
In other words, you have options. Your educational journey can — and should — be unique to you, constructed around your individual needs and preferences. Before you start planning your path, take a few moments to answer the below questions, and gain a better understanding of your particular educational needs and preferences.
- Education: How do you learn best? Can you learn on your own, or do you prefer an instructor-led course?
- Situation: Can you accommodate a full-time schedule, or do you need part-time flexibility? Are you able to do an in-person class or do you require online training?
- Finances: How much are you willing to pay for your education?
- Time: How long are you willing to invest in learning the trade? Can you afford to take off a few months, or would a part-time schedule over a longer period of time work better for you?
- Plan: What’s your projected timeframe for obtaining new skills and re-entering the job market?
Keep your answers handy as you review the educational paths laid out below!
College Degree
It might not be necessary to get a college degree before becoming a data analyst, but it sure is helpful to have one!
A traditional four-year degree will equip you with both the practical skills and theoretical knowledge that you’ll need to thrive in data analytics. The education provided at colleges is often a great deal more comprehensive than those of intensive workshops and self-study.
The benefits extend far beyond formal classwork, too; by attending college, you’ll have the opportunity to build a valuable network of peers, professors, and industry professionals. Conveniently, many degrees also culminate in a capstone project, which can serve as the centerpiece of your entry-level portfolio and showcase your technical capabilities to potential employers.
Employers may call for data analysts to have a four-year degree in one of the following disciplines:
- Business Information Systems
- Economics
- Computer Science
- Information Management
- Mathematics
- Statistics
That said, data analysts aren’t required to have a degree in one of these fields — or at all! (Don’t worry; we’ll go more into that later.)
You can become a data analyst with any degree as long as you demonstrate that you have the necessary proficiencies. If you already earned your undergraduate marks in a different field, you may want to consider investing in a boot camp or self-directed program that will allow you to pick up any practical skills that you may be missing.
All this said, the college track isn’t right for everyone. It requires an investment of time and money and, given that most institutions are structured on a full-time schedule, you must be sure that you can accommodate classes around your personal or professional obligations.
Boot Camps
Data analytics boot camps are short-term, intensive learning programs that equip you with all the in-demand skills you need to enter the job market. These intensive courses are known for their flexibility and accessibility, can be held online or in-person, and usually wrap up within three to six months.
When compared to a degree program, boot camps require a shorter time and cost commitment. Data analytics boot camps also provide essential training in on-the-job skills such as data mining, data preparation, behavioral analytics, machine intelligence, and specific software.
Attendees often graduate with these practical capabilities — but, on the flip side, may not receive the same comprehensive theoretical background that a college graduate might get. However, it is worth noting that the resulting lack of theoretical training often doesn’t worry potential employers.
According to a 2017 study from Indeed, 72 percent of employers see boot camp grads as equally prepared to be high performers as conventional degree holders, while 12 percent believe that boot camp grads are “more prepared and more likely to be high performers.” In fact, 80 percent of surveyed employers have hired boot camp grads, and almost all (99.8 percent) said they would hire one again.
Moreover, data from Course Report’s 2018 survey on coding boot camp alumni outcomes indicates that the majority of boot camp grads find full-time jobs. Nearly 80 percent of grads surveyed say that they have been employed in a position that requires them to use skills learned at a boot camp.
Interested? For more information on what a real boot camp program can offer, check out UT Austin’s Online Data Analytics Boot Camp curriculum. This 24-week intensive boot camp program provides broad coverage of the most in-demand tech, including Excel, SQL, Tableau, and more.
Self-Directed Learning
Solo learning has never been so easy. Today, tutorials, digital textbooks, and self-guided courses can be accessed with a quick Google search.
It’s hard to beat the low cost, flexibility, and sense of independence that embarking on a self-directed education creates. You can develop your own curriculum, set your own schedule, and learn according to your interests and goals.
Self-directed learning can offer an adaptable, budget-friendly means of picking up practical skills — but it comes with a few caveats.
Unlike college programs or boot camps, independent study programs don’t come with a learning plan or supportive instructors. Yes, that absence allows you to develop your own curriculum, but it also places the responsibility of doing so on you. You need to be able to hold yourself accountable and keep pushing yourself to learn. If you don’t, you may find that you aren’t picking up the skills you need quickly (or at all).
This route is only recommended to those who are extremely self-motivated. Be honest with yourself! Can you handle the responsibility? If you tend to need support or guidance — and let’s be honest, most people do — a boot camp or formal program might be a better fit.
It’s also important to note that because you won’t emerge from a self-led course with a degree or certificate, you will need to develop a stand-out portfolio to convince employers that you have the skills necessary to do the job you’re applying for.
Alternatively, self-directed forays into data science could be your start to a more long-term, committed program. Many non-tech learners use self-guided learning strategies to build a foundational understanding of data analysis before enrolling in formal programs.
Looking for ideas of where to start your self-guided education? We have you covered!
Best Books
Who doesn’t love a good book? If you’re new to data analytics, these top-tier texts can help you gain a foundational understanding of basic data science principles.
- Data Analytics Made Accessible — by Dr. Anil Maheshwari. A deep dive into data mining, visualization, neural networks, and other relevant topics.
- Data Science for Dummies — by Lillian Pierson. A beginner-friendly resource that focuses on the business side of data analytics and provides guidance on how to enter the field.
- Big Data: A Revolution That Will Transform How We Live, Work, and Think — by Viktor Mayer-Schönberger and Kenneth Cukier. An overview of how big data has shaped industries as diverse and essential as business, government, medicine, and science.
Best Magazines and Trade Publications
Following the right blogs and news sources can help to keep your interest in data science alive, especially during motivational slumps.
- Analytics Insight — A print magazine that shares big data news and trends as well as interviews, articles,and commentary on current happenings in the field.
- Datafloq — An online magazine that provides insights into emerging technologies such as big data, blockchain, and AI.
- Database Trends and Applications — An online resource that provides news, analysis, whitepapers, webinars, and more.
Best Video Learning Channels
Are your eyes sore from reading yet? Video-based data science tutorials make it easier to refine both your knowledge and interest in the field.
- The Semicolon — Provides simple explanations of complex topics such as machine learning, Python, and (most importantly) data analytics.
- Data School — Offers in-depth tutorials on how to use Python for data science.
- David Langer — Industry expert Dave Langer provides an abundance of high-quality tutorials on topics relating to data science.
Improve Your Hiring Chances
At the end of the day, employers are just as hopeful as you are that you’re the perfect fit for the role; however, they want to minimize turnover risk and cost by choosing the best candidate possible. Every position you apply for will probably attract dozens of qualified candidates, all of whom want the role at least as much as you do.
Demonstrating passion, interest, and competence is a crucial part of setting yourself apart from the crowd. To do so, you’ll have to dedicate time and effort toward polishing your resume, compiling your portfolio, practicing for interviews, and building a supportive professional network.
Not sure where to start? Check out the resources below for tips on how to prepare yourself for the application process!
- What Every Aspiring Data Scientist Needs to Know About Networking — Towards Data Science. Provides tips on how up-and-coming data analysts can make the most of their professional networks.
- Data Analyst Resume: Guide & Examples for 2020 — Career Blog. Explains how to present yourself in the best light possible through resume optimization.
- How to Build a Data Science Portfolio — Towards Data Science. Describes what type of projects can be included in your portfolio (and how you can potentially skate by the experience requirement).
- Data Analyst Interview Questions: Show Off Your Experience — Dice Insights. Explains what new data analysts can expect from a job interview and provides tips on how to impress their interviewers.
How to Break Into Your Chosen Industry
All data analysts will have the same core set of professional skills. However, if you already know which industry you hope to work in once you finish your education and enter the job market, you can tailor your capabilities and appeal to potential employers in that field.
With this in mind, let’s revisit the industries we spotlighted at the beginning of this article and explain what you can do to optimize your hard and soft skill sets for your dream job.
Retail
To thrive in retail, you’ll need to have strong technical skills and even better communication capabilities. You will also need to be comfortable generating and analyzing large sets of data for internal company stakeholders via SQL and NoSQL queries.
Because you will likely need to communicate your findings with both clients and company directors, you should be able to clearly explain how the insights you see illustrate consumer trends and feed into retail strategies.
Because retail is often focused on visual appeal and branding, you may benefit from placing your portfolio projects on an interactive, visually pleasing site for employers to review.
Finance
Data analysts in the financial world need to develop their critical thinking capabilities and problem-solving skills. Employers will also likely require a comprehensive understanding of SQL, knowledge of various data models, and demonstrable proficiency in Excel.
You should be able to generate corporate reports. Prospective financial data analysts should be able to retrieve data from diverse environments, including but not limited to: websites, emails, company logs, and even face-to-face client interactions.
Manufacturing
Data analysts who want to build a career in the manufacturing industry must be highly detail-oriented and deadline-aware. They should have an advanced understanding of Excel and relational databases. Foundational knowledge of predictive analytics is also a must, given that data analysts in manufacturing often focus on calculating when systems will require intervention.
Analysts in manufacturing should value quality and accuracy, and be able to communicate equally well with team members, business leaders, and non-technical colleagues.
Healthcare
Most data analysts in the healthcare field have some previous background in medicine. You’ll need to demonstrate a deep understanding of common healthcare procedures; many jobs will require specific certifications.
Employers want job candidates who have excellent problem-solving skills and proficiency in managing medical records. Health care data analysts should also have experience in both spreadsheet analysis and visualization methods.
Transportation
Aspiring data analysts who want to break into the transportation industry should have a familiarity with data visualization, programming, statistical analysis, and econometric modeling. Experience and/or demonstrated interest in urban planning and policy research can also be beneficial.
Analysts in transportation should also have excellent communication and collaboration skills, as their role will almost certainly be in a project-based or consulting environment.
So, What Now?
Now that you’ve come this far, you know how to become a data analyst and make your mark in any industry.
Meet the challenge head-on! Take some time, right now, to figure out which educational path works best for you and what you can do to take the first step. You can change your life and build the data analytics career of your dreams.